Due to their enormous surface region and moisture absorption capability, textile materials allow bacteria to flourish, resulting in unfavorable impacts on the textile and end-users according to the latest study published in the journal coatings.
Study: A Supervised Machine-Learning Prediction of Textile's Antimicrobial Capacity Coated with Nanomaterials. Image Credit: Anna Aybetova/Shutterstock.com
Researchers introduce innovative regression models for predicting the antibacterial activity of nano-textiles after many continuous standard washes.
Microbial Actions on Textile Materials
Textile fabrics are prone to bacterial infiltration due to their intrinsic qualities in conjunction with various environmental conditions such as moisture and temperature. The emergence of hazardous bacteria affects the textile, such as producing toxic fumes, spots, and staining, and an increased likelihood of transmission to the consumer. It is critical to apply antimicrobial treatments to textile materials to reduce the development of bacteria while they are in use.
Microbes may proliferate and persist on textiles for days to months. The colonization of microbial pathogens on surfaces results in the creation of biofilms that are impenetrable to antimicrobial treatments.
Antimicrobial Nanomaterials
Antimicrobial nanomaterials (NMs) are used in combination with antibiotics to prevent the growth of multidrug-resistant bacteria and give a multipurpose alteration to textiles. NMs enhance fabric tolerance to microorganisms, boost dye absorbency, and modify hydrophilicity, enabling for effective synthesis process without affecting textile characteristics.
The antibacterial properties of NMs (organic and inorganic) are connected to microbes' incapacity to development of resistance to the latter. Many organic and inorganic NMs have been used in the textile industry, including inorganic metal and metal oxide NMs such as silver (Ag) and titanium oxide.
The antibacterial mechanisms of NMs entail several types of action. The key routes include the generation of Reactive Oxygen Species (ROS), the rupture of microbial cell membranes, and the discharge of chemicals that restrict the life functionality of microorganisms, inevitably leading to their death.
Many parameters, including deposition process and washing circumstances, influence the antibacterial capabilities of fabrics treated with NMs (nano-textiles). Depending on the kind of NM and the fiber, there are many ways for depositing NMs on textiles.
Nanomaterials are either integrated into the fibers after extrusion or adhered to the surface during processing. Textiles are subjected to a variety of conditions over their lifetime, including laundering, warmth, and dry cleaning; in some cases, it is critical to know how well the textile can retain its antimicrobial qualities.
Machine Learning Tool for Textile Microbial Activity
Machine learning (ML) is a subfield of artificial intelligence (AI) covering a broad range of modeling techniques used for varied data computational requirements; it has gained traction in the past few years across several scientific fields.
In this study, researchers used regression models to estimate antimicrobial capacity as a zone of inhibition of several NMs. A machine learning approach is provided to predict the antibacterial efficacy and durability of nano textiles after numerous washings. As inputs, this method estimates antimicrobial capacity by examining the p-chem characteristics of NMs, exposure settings, and bacterium species.
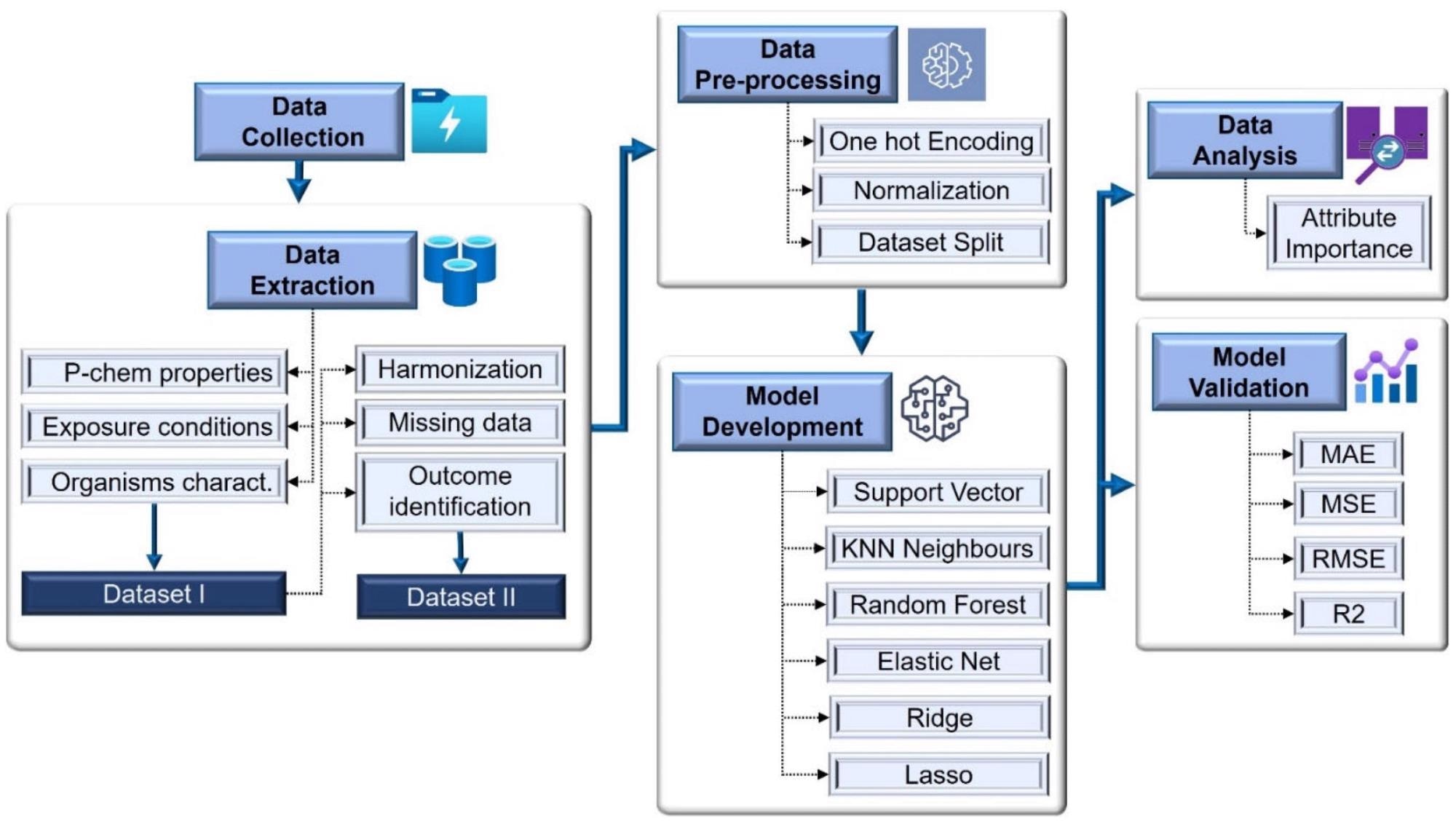
Model development workflow. We employed different regression models. Evaluated by mean absolute error (MAE), mean square error (MSE), root mean square error (RMSE), and coefficient of determination or R-squared (R2).
Regression Models
In this work, the regression model discovered a relationship between NMs' p-chem characteristics and experimental settings and the proportion of bacteria decrease, allowing for the forecast of nano-textile antimicrobial durability after numerous washes. Various regression methods were used as potential nominees to determine which model provided the best accurate forecast. These models include LASSO, RR, ENR, RF, SVR, and KNN.
Research Findings
The organisms (bacteria and fungus) were divided into 18 separate categories.
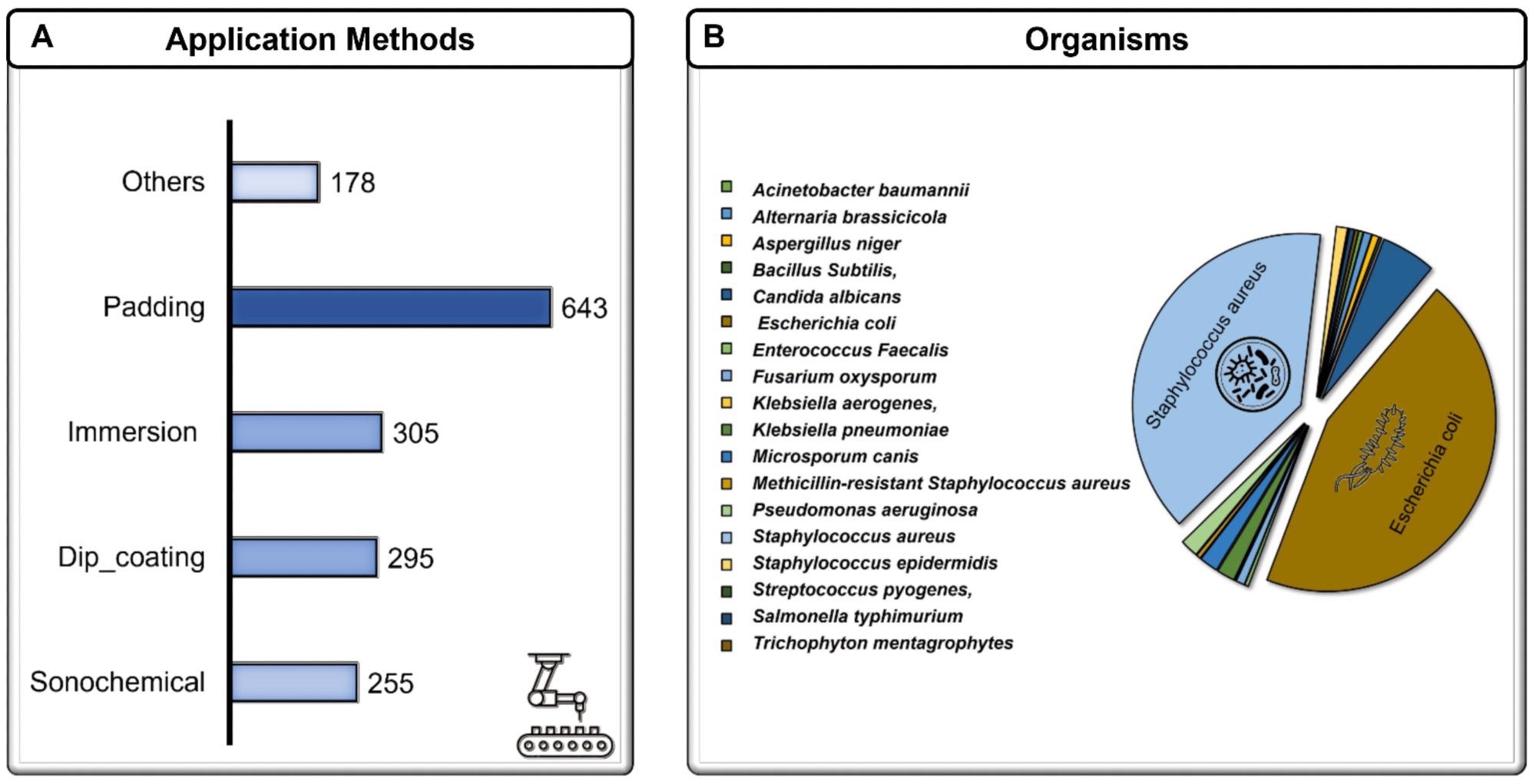
A) Different application methods; (B) different investigated organisms (bacteria and fungus) used as input.
We discovered that RF surpassed the others based on the assessment and validation methodologies. To sustain and retain the antibacterial capabilities of nano textiles, robust adherence between NMs and surfaces is necessary.
The most critical factor determining the efficiency of antimicrobial nano textiles is the kind of NM. The NM diameter, type of surface, assessment technique, and washing cycles were determined as significantly relevant parameters, followed by the application (deposition) technique, morphology, and endurance test. The effects of NM content, period, adhesive type, and species subjected had a relatively minor impact on the forecast of the antibacterial ability of nano-textiles.
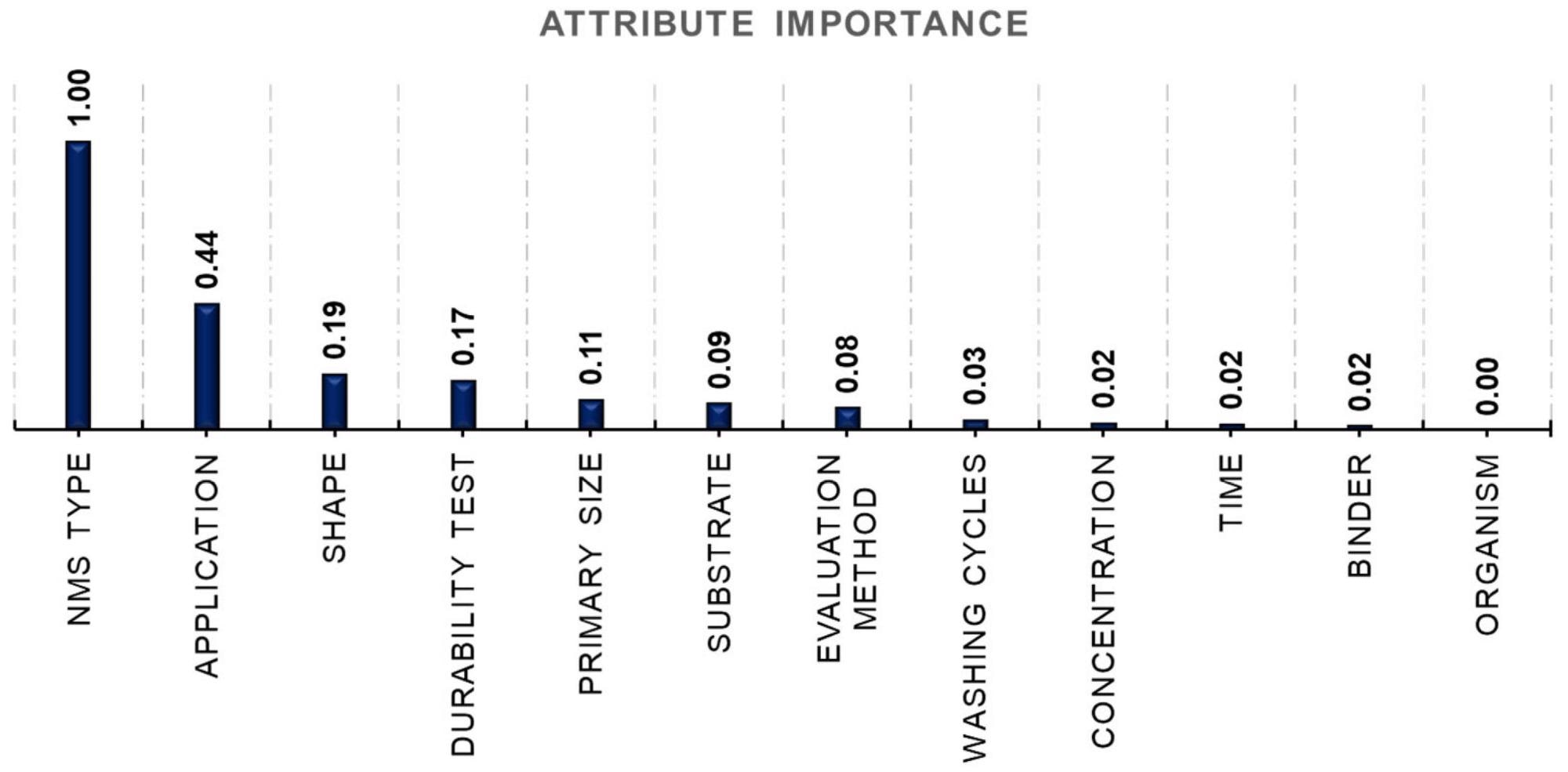
Figure 3. Attribute importance analysis by RF model.
In short, the machine learning (ML) technique that forecasted nanotextile bactericidal capability before and after multiple washes (up to 50 washes) washes to determine the most relevant factors that impact the forecast was implemented successfully. The capacity to predict antimicrobial capabilities of nano-textiles, particularly in hospital contexts, holds immense promise for offering concrete therapeutic advantages.
Continue reading: Nano-based Textiles and Fabrics: An Overview
Reference
Mirzaei, M., Furxhi, I., Murphy, F. & Mullins, M., (2021) A Supervised Machine-Learning Prediction of Textile's Antimicrobial Capacity Coated with Nanomaterials. Coatings, 11(12). 1532. Available at: https://www.mdpi.com/2079-6412/11/12/1532
Disclaimer: The views expressed here are those of the author expressed in their private capacity and do not necessarily represent the views of AZoM.com Limited T/A AZoNetwork the owner and operator of this website. This disclaimer forms part of the Terms and conditions of use of this website.