In a recent article in Nature Communications, researchers presented a new approach to anticounterfeiting using fluorescent nanodiamonds (FNDs) as physical unclonable functions (PUFs). The study introduces a three-dimensional encoding scheme that increases the encoding capacity of PUF labels, combined with a deep metric learning algorithm for improved authentication. This approach aims to address challenges in counterfeiting.
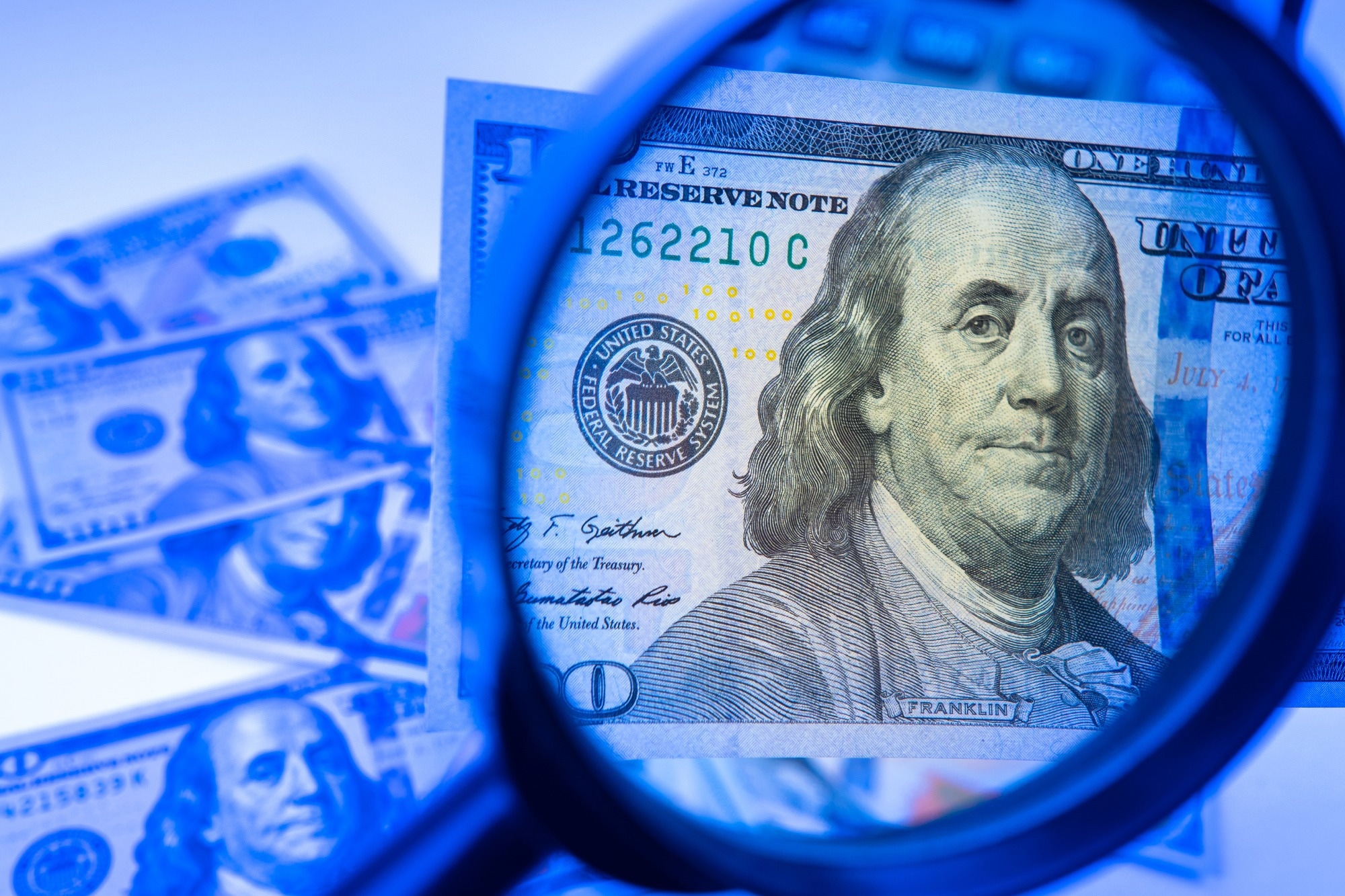
Image Credit: FOTOGRIN/Shutterstock.com
Background
Counterfeiting has become a pervasive issue, affecting not only luxury goods but also pharmaceuticals and electronics. The economic impact of counterfeit products is staggering, with estimates suggesting billions in losses annually.
PUFs have gained attention as a promising technology for secure authentication due to their inherent uniqueness and difficulty in replication. FNDs, in particular, are recognized for their optical properties, which enable high-dimensional encoding. However, existing PUF systems often face challenges related to reproducibility and noise interference, limiting their practical applications.
The authors of this study aim to overcome these limitations by developing an advanced encoding and authentication method that takes advantage of the unique characteristics of FNDs.
The Current Study
The experimental setup included a customized wide-field fluorescence microscope for high-resolution imaging of the FND PUF labels. A continuous 532 nm laser was used as the excitation light source, with polarization controlled by a half-wave plate and a polarizer, allowing precise manipulation of the laser's polarization direction. Fluorescence emitted from the FNDs was captured by a water-cooled EMCCD camera, providing a field of view of approximately 30 × 30 μm.
The fabrication process for the FND PUF labels included plasma treatment of cover slides to enhance surface properties, followed by immersion in a 3-aminopropyltriethoxysilane (APTES) solution to create a positively charged surface. Carboxylated FNDs were then drop-cast onto the treated slides, incubated for electrostatic absorption, and covered with a protective polydimethylsiloxane (PDMS) layer.
To digitize the fluorescence images, a contrast measurement system was established, correlating photon counts to pixel contrast values. Nine distinct contrast levels were defined to represent the encoded information. The images were analyzed using a convolutional neural network (CNN) designed for metric learning, enabling the system to differentiate between various PUF labels effectively.
Results and Discussion
The results demonstrated the efficacy of the proposed three-dimensional encoding scheme. The authors successfully distinguished all 300 PUF labels within a defined threshold range, showcasing the robustness of their method. The authentication process revealed high reproducibility, with similarity indexes calculated among multiple digitized images of the same PUF label. The findings indicated that the system maintained a consistent performance even under varying conditions, such as noise interference and long-term stability.
The deep metric learning framework was crucial in enhancing the authentication process. By training the CNN on pairs of digitized images, the system learned to effectively identify and differentiate between similar and dissimilar labels. The heat maps generated from the similarity scores provided visual insights into the authentication results, illustrating the system's ability to accurately classify PUF labels based on their encoded information.
The study also highlighted the advantages of using FNDs in anticounterfeiting applications. The unique optical properties of FNDs allowed for high-dimensional encoding, significantly increasing the information capacity compared to traditional methods. The authors also discussed the potential for integrating this technology into various industries, emphasizing its scalability and adaptability.
Conclusion
This study presents the use of fluorescent nanodiamonds as physical unclonable functions for anticounterfeiting. The development of a three-dimensional encoding scheme, combined with a deep metric learning algorithm, addresses the challenges of reproducibility and noise interference that have affected previous PUF systems. The results show that this approach can provide a reliable and scalable solution for product authentication across various sectors.
As counterfeiting remains a global issue, the integration of such technologies could help improve product integrity. The authors' work provides a foundation for further research and development in anticounterfeiting strategies.
Journal Reference
Wang L., et al. (2024). High-dimensional anticounterfeiting nanodiamonds authenticated with deep metric learning. Nature Communications. DOI: 10.1038/s41467-024-55014-2, https://www.nature.com/articles/s41467-024-55014-2