Therapeutic and drug delivery systems built with nanomaterials contain molecules ranging in size. Due to developments in lipid nanoparticle (LNP) technology, the first SARS-CoV-2 (COVID-19) vaccines built with siRNA therapy and mRNA delivery systems were formed.
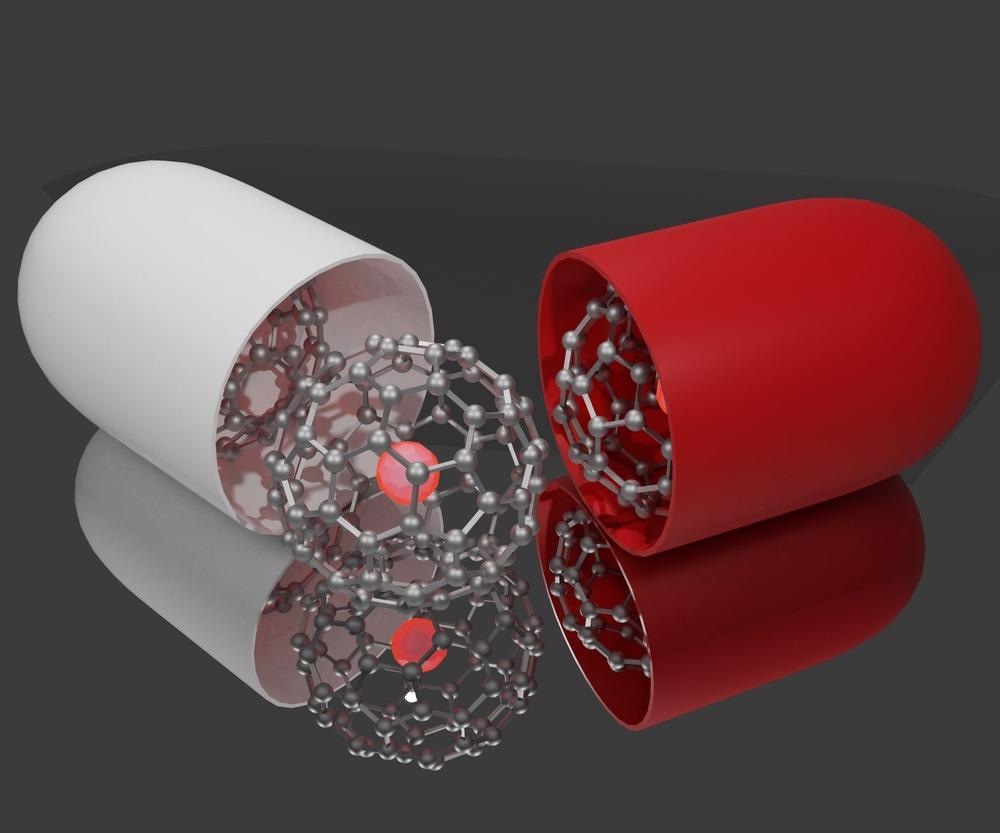
Image Credit: Love Employee/Shutterstock.com
Although LNPs, as well as other nanoparticles, have demonstrated use in stabilizing and delivering cargo to target areas, targeted nanomedicine remains difficult to achieve in practice.
There is now a significant divergence between fundamental science and translational progress in the field of nanomedicine.
To advance this field, researchers will need to overcome the barrier that prevents clinical applications of nanomaterial systems.
By designing strategies and methodologies that aim to investigate the therapeutic interactions between these nano-based technologies and biological systems, significant progress can be made in the field of nanomedicine. This idea is illustrated in Figure 1.
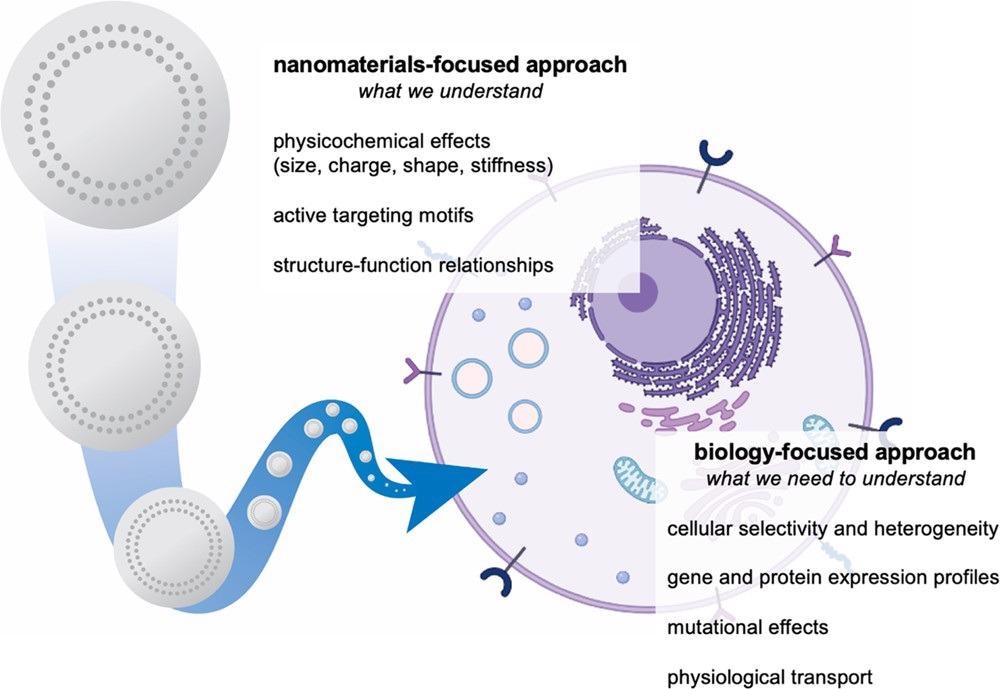
Figure 1. Development of nanomaterials systems for drug delivery is traditionally focused on the study and optimization of materials properties. To overcome biological delivery barriers, we must shift the focus to understanding the interactions of cells and tissues with nanocarriers. We can achieve this through integrated approaches, including the use of nanoparticle libraries, pooled screening, and omics characterization. Image Credit: Boehnke and Hammond, 2022
The introduction and increased accessibility of high-throughput sequencing methods has accelerated the field of therapeutic genomics. As shown in Figure 2, these essential concepts are starting to be used in nanomedicine, presenting an unprecedented potential to develop the discipline.
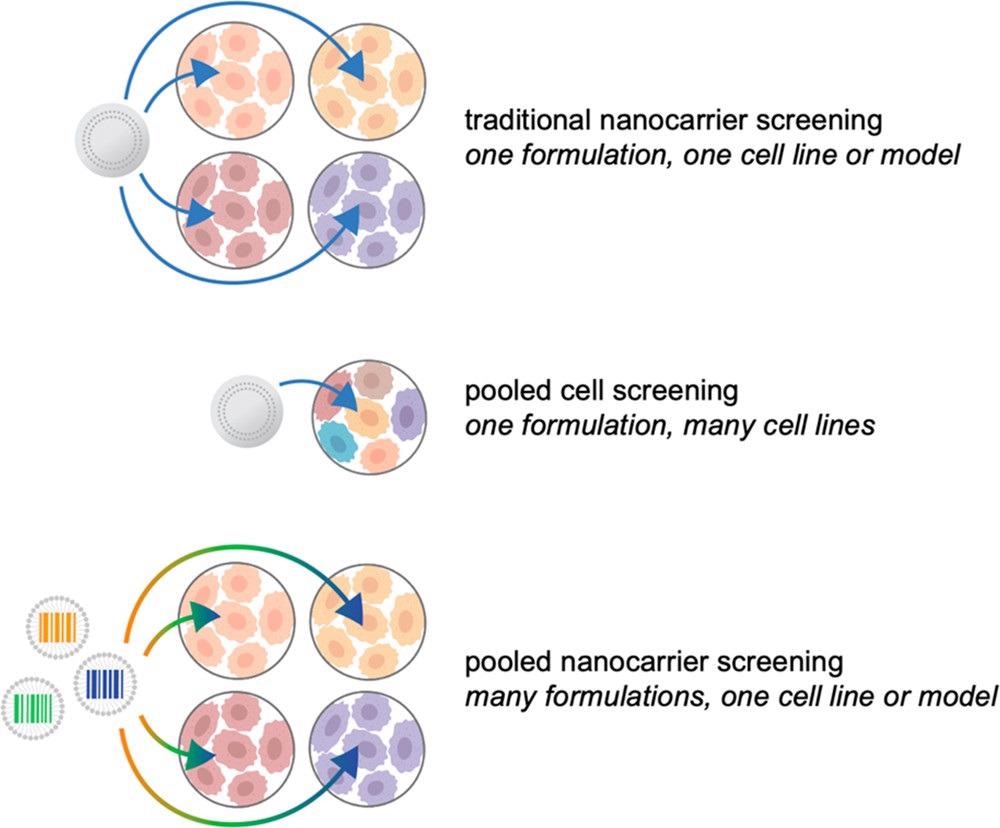
Figure 2. Illustrated examples of nanocarrier screening approaches. Traditionally, candidate formulations are tested iteratively, in one or two models at a time, with a focus on materials property testing. Through the use of pooled cell screening, the same formulations can be screened against hundreds of cell lines simultaneously, providing insight into the biological features mediating successful nanocarrier targeting and uptake. Alternatively, barcoding strategies can be implemented to pool nanocarriers for accelerated biological screening. Image Credit: Boehnke and Hammond, 2022
Advancements in Nanocarriers
Extensive, combinatorial libraries of lipid and polymer-based nanocarriers for gene and drug delivery applications have resulted from advancements in nanocarrier synthesis, including lipid nanoparticles for RNA transport, chemically varied core–shell NPs, and lipocationic polyesters.
Research has shown that successful transfection is dependent on three key factors, each of which is controlled by different physicochemical features of the nanocarrier: RNP uptake (protonation state), hydrophobicity, and cellular toxicity (polyplex diameter).
One method for swiftly screening a large number of drug delivery vehicles in a single system is to use barcoded nanocarriers. Early reports of barcoded nanoparticles depended on imaging-based decoding algorithms with restricted clinical implications, and nanocarrier barcoding strategies have come a long way.
In addition, DNA barcodes have been integrated into liposomes in combination with small molecule therapies to analyze both delivery success and therapeutic efficacy collectively manner. The efficacy of small molecule delivery can be assessed using this method by combining treatment response (e.g., viability) with the number of DNA barcodes per cell.
While this research offers a simple way to link liposome delivery to treatment success, it also exposes the limitations of nucleic-acid-based barcodes.
Pooled screening has begun to illustrate the power of DNA barcodes in accelerating nanocarrier development.
Alternative barcoding systems, particularly those that enable direct nanocarrier tracking in vivo and are compatible with various drug delivery vehicles, are urged to be considered by the field.
To deposit layers of operational polyelectrolytes on a charged surface, layer-by-layer (LbL) assembly can be used. Besides therapeutic delivery, the modular structure of LbL assembly allows for basic research into the impacts of specific nanocarrier parameters, as demonstrated in Figure 3.
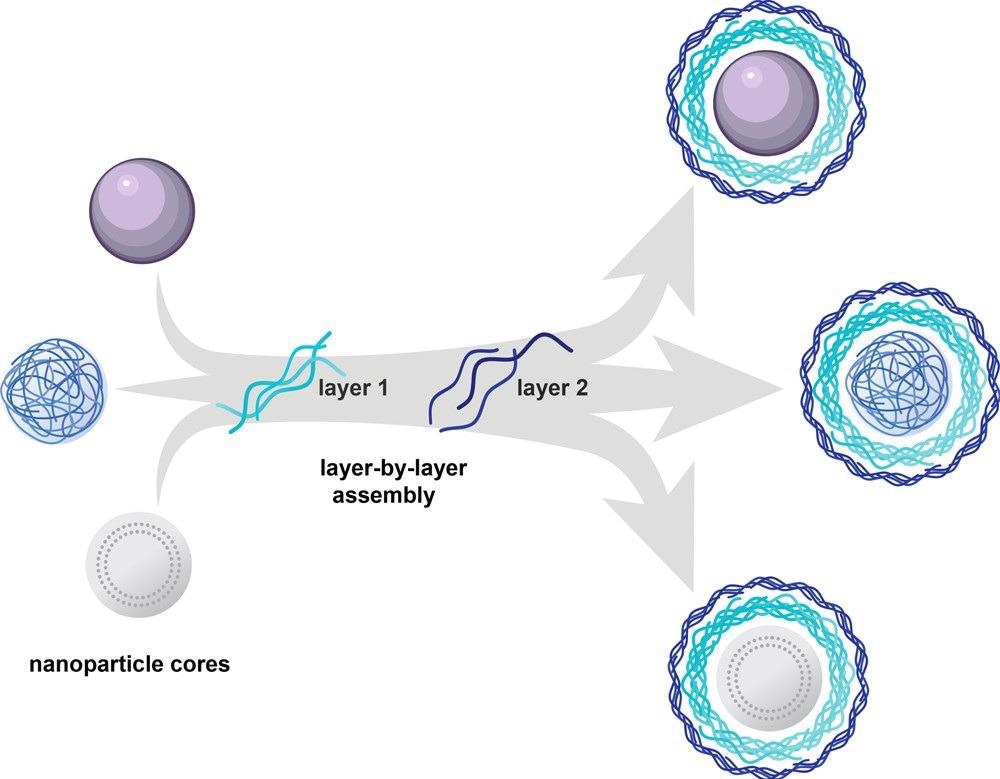
Figure 3. Layer-by-layer assembly can be used to electrostatically coat a wide range of nanoparticle cores with functional polyelectrolytes. The approach enables complete coating of the carrier core and thus decouples the outer layer functionality from physical or chemical characteristics of the core. Image Credit: Boehnke and Hammond, 2022
Figure 4 shows how LbL assembly is used to develop NP libraries to assess new designs with tumor-targeting properties.
Novel surface chemistries with distinct intracellular transport properties and remarkable affinity for ovarian cancer cells over non-neoplastic cells have been identified using this strong NP screening technique.
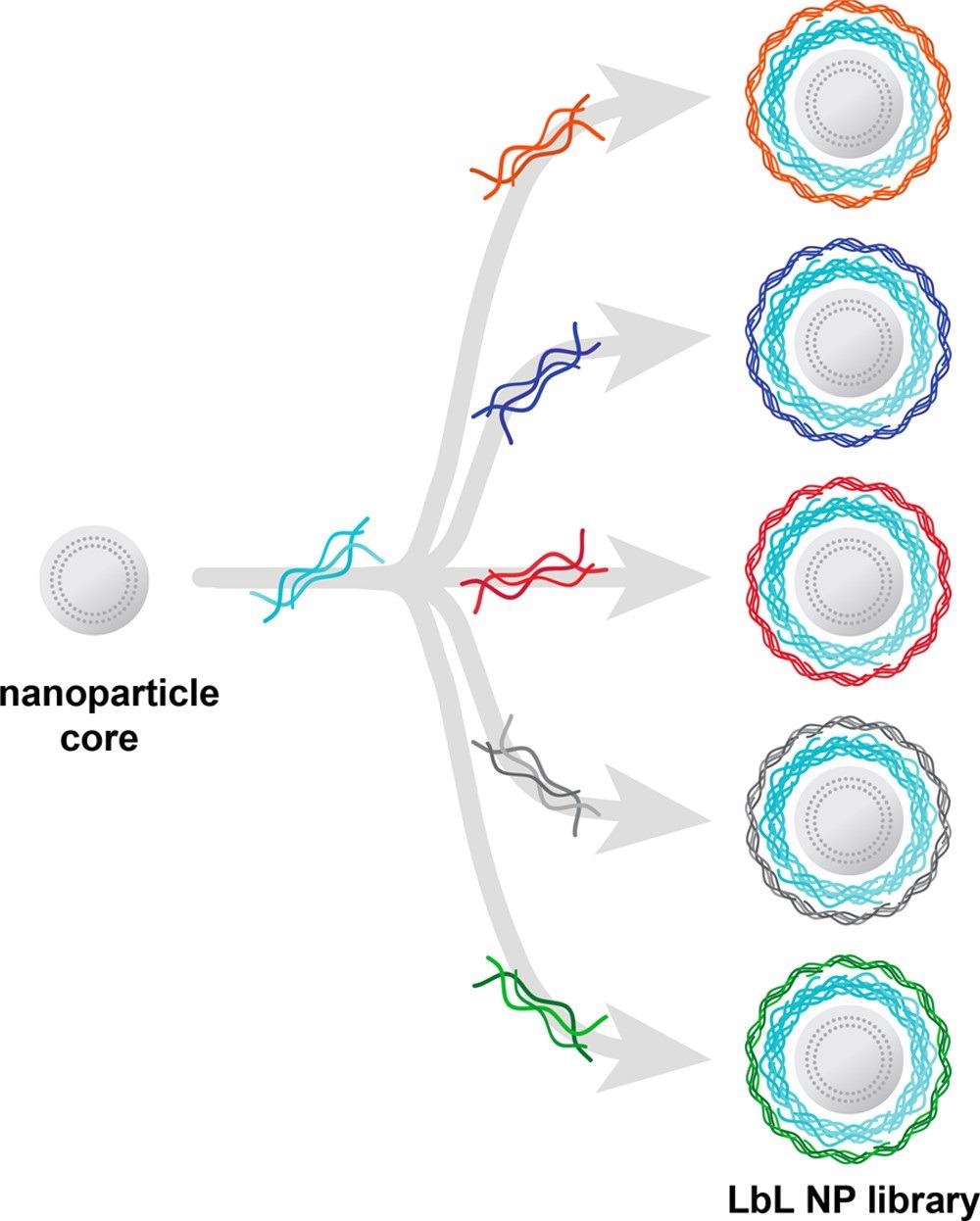
Figure 4. Layer-by-layer assembly enables the generation of nanocarrier libraries wherein one component is varied while all others are kept constant. Illustrated here is an example of a common nanoparticle core and polyelectrolyte layer being separately coated with a range of polyanions to generate a nanocarrier library focused on evaluating surface chemistry effects. Image Credit: Boehnke and Hammond, 2022
Results and Discussion
Researchers believe that overcoming existing challenges will require two synergistic strategies: (1) thorough in vitro screens to help understand key cellular qualities resulting in nanocarrier therapeutic success, and (2) the advancement and use of adequate, relevant models to relate cellular and tissue-specific nanocarrier selectivity with effective circulation and trafficking properties.
Because of biological heterogeneity, there are various challenges limiting the successful translation of new therapeutic agents in the field of small molecule drug discovery.
Restricted preclinical screens that fail to grasp the diversity and complexity of human patients are often blamed for the absence of reproducible data and failing to reproduce efficacy beyond basic models.
A lack of appropriate preclinical models to completely assess the therapeutic efficacy of nanomedicine has been proven. Models should be able to accurately depict human complexity and disease, as well as anticipate response to tested treatments.
By shielding encapsulated cargo from degradation, extending circulation duration, and reducing harmful side effects, the development of nanocarriers for therapeutic delivery applications has already resulted in substantial clinical improvements.
Translational successes in targeted medication delivery, on the other hand, have been very limited.
The continuous development and utilization of relevant preclinical models, such as multicellular organoids and mice models capable of replicating human immunological responses, will be required to further enhance the current understanding of the nano-bio interface.
Finally, future research will be able to holistically discover the critical characteristics influencing efficient cell targeting and uptake, from both materials and biology perspectives, by comprehensively combining these methodologies, as illustrated in Figure 5.
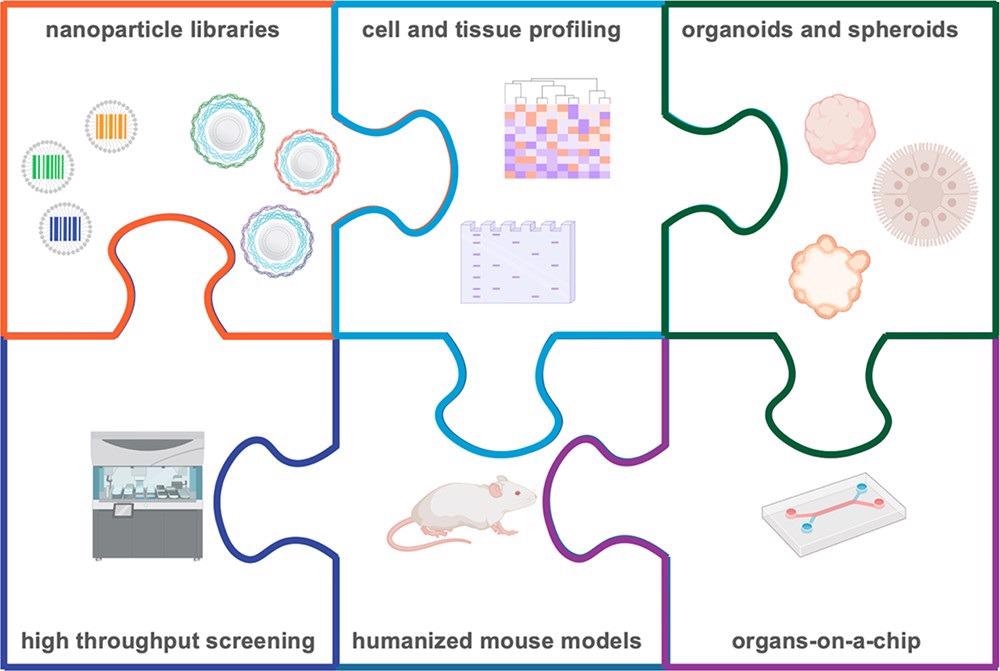
Figure 5. Informed nanocarrier design is possible through the use of nanoparticle barcodes, pools, and libraries, which can be interfaced with high throughput screening, biological profiling, and physiological in vitro and in vivo models. Through these integrated approaches, we can gain a more thorough understanding of the biological characteristics necessary for successful delivery of therapeutics. Image Credit: Boehnke and Hammond, 2022
Conclusion
Since there is presently a significant gap between in vitro and in vivo nanocarrier performance due to a lack of mechanistic knowledge into the causes of these failures, physiologically representative models must be developed and implemented to allow translation of suitable candidates recognized through in vitro screens.
Developing and testing customized mouse models is often beyond the scope of nanotechnology-focused research teams. As a result, researchers should form partnerships with biological and clinical researchers to benefit from their knowledge in disease-focused preclinical testing.
By providing scientists and physicians with the tools given by nanomedicine to tackle unaddressed difficulties, these interdisciplinary methods also pave the way for a successful nanomedical future.
Continue reading: Manifesting Multidisciplinary Nanomedicine Research with the MMS
Journal Reference:
Boehnke, N., & Hammond, P. T. (2022) Power in Numbers: Harnessing Combinatorial and Integrated Screens to Advance Nanomedicine. JACS Au, 2(1), pp. 12–21. Available Online: https://pubs.acs.org/doi/10.1021/jacsau.1c00313.
References and Further Reading
- Barberio, A. E., et al. (2020) Cancer Cell Coating Nanoparticles for Optimal Tumor-Specific Cytokine Delivery. ACS Nano, 14, p. 11238. doi.org/10.1021/acsnano.0c03109.
- Boehnke, N., et al. (2020) Electrostatic Conjugation of Nanoparticle Surfaces with Functional Peptide Motifs. Bioconjugate Chemistry, 31(9), pp. 2211–2219. doi.org/10.1021/acs.bioconjchem.0c00384.
- Kong, S. M., et al. (2021) Stiffness of targeted layer-by-layer nanoparticles impacts elimination half-life, tumor accumulation and tumor penetration. Processing of the National Academy of Sciences of the USA, 118, p. e2104826118. doi.org/10.1073/pnas.2104826118.
- Seyhan, A A (2019) Lost in translation: the valley of death across preclinical and clinical divide - identification of problems and overcoming obstacles. Translational Medicine Communications, 4, p. 18. doi.org/10.1186/s41231-019-0050-7.
- Sayed, N., et al. (2020) Clinical trial in a dish using iPSCs shows lovastatin improves endothelial dysfunction and cellular cross-talk in LMNA cardiomyopathy. Science Translational Medicine, 12(554), p. eaax9276.doi.org/10.1126/scitranslmed.aax9276.
- Lam, C K & Wu, JC (2021) Clinical Trial in a Dish Using Patient-Derived Induced Pluripotent Stem Cells to Identify Risks of Drug-Induced Cardiotoxicity. Arteriosclerosis, Thrombosis, and Vascular Biology, 41(3), pp. 1019–1031. doi.org/10.1161/ATVBAHA.120.314695.
- Barretina, J., et al. (2019) The Cancer Cell Line Encyclopedia enables predictive modelling of anticancer drug sensitivity. Nature, 565(7738), pp. E5– E6. doi.org/10.1038/nature11003.
- Barretina, J., et al. (2012) The Cancer Cell Line Encyclopedia enables predictive modelling of anticancer drug sensitivity. Nature, 483, pp. 603–607. doi.org/10.1038/nature11003.
- Ghandi, M., et al. (2019) Next-generation characterization of the Cancer Cell Line Encyclopedia. Nature, 569(7757), pp. 503–508. doi.org/10.1038/s41586-019-1186-3.
- Li, H. X., et al. (2019) The landscape of cancer cell line metabolism. Nature Medicine, 25(5), pp. 850–860. doi.org/10.1038/s41591-019-0404-8.
- Dharia, N. V., et al. (2021) A first-generation pediatric cancer dependency map. Nature Genetics, 53(4), pp. 529–538. doi.org/10.1038/s41588-021-00819-w.
- Corsello, S. M., et al. (2020) Discovering the anticancer potential of non-oncology drugs by systematic viability profiling. Nature Cancer, 1, p. 235. doi.org/10.1038/s43018-019-0018-6.
- Sinha, R., et al. (2021) A pan-cancer survey of cell line tumor similarity by feature-weighted molecular profiles. Cell Reports Methods, 1, p. 100039. doi.org/10.1016/j.crmeth.2021.100039.
- Yu, C. N., et al. (2016) High-throughput identification of genotype-specific cancer vulnerabilities in mixtures of barcoded tumor cell lines. Nature Biotechnology, 34(4), pp. 419–423. doi.org/10.1038/nbt.3460.
- Jin, X., et al. (2020) A metastasis map of human cancer cell lines. Nature, 588(7837), pp. 331–336. doi.org/10.1038/s41586-020-2969-2.
- Boehnke, N., et al. (2021) Massively parallel pooled screening reveals genomic determinants of nanoparticle-cell interactions. bioRxiv. doi.org/10.1101/2021.04.05.438521.
- Lazzari, G., et al. (2017) Multicellular tumor spheroids: a relevant 3D model for the in vitro preclinical investigation of polymer nanomedicines. Polymer Chemistry, 8(34), pp. 4947–4969. doi.org/10.1039/C7PY00559H.
- (2018) Method of the Year 2017: Organoids. Nature Methods, 15, p. 1.doi.org/10.1038/nmeth.4575.
- (2021) The promise of organoids and embryoids. Nature Methods, 20(2), p. 121.doi.org/10.1038/s41563-021-00926-3.
- Drost, J & Clevers, H (2018) Organoids in cancer research. Nature Reviews Cancer, 18(7), pp. 407–418. doi.org/10.1038/s41568-018-0007-6.
- Pauli, C., et al. (2017) Personalized In Vitro and In Vivo Cancer Models to Guide Precision Medicine. Cancer Discovery, 7(5), pp. 462–477. Available online: https://cancerdiscovery.aacrjournals.org/content/7/5/462.
- Jenkins, R. W., et al. (2018) Ex Vivo Profiling of PD-1 Blockade Using Organotypic Tumor Spheroids. Cancer Discovery, 8(2), pp. 196– 215. Available online: https://cancerdiscovery.aacrjournals.org/content/8/2/196.
- Shi, J. J, et al. (2017) Cancer nanomedicine: progress, challenges and opportunities. Nature Reviews Cancer, 17(1), pp. 20–37.doi.org/10.1038/nrc.2016.108.
- Mitchell, M. J, et al. (2021) Engineering precision nanoparticles for drug delivery. Nature Reviews Drug Discovery, 20, pp. 101–124. doi.org/10.1038/s41573-020-0090-8.
- Tran, S., et al. (2017) Cancer nanomedicine: a review of recent success in drug delivery. Clinical and Translational Medicine, 6(1), p. e44, doi.org/10.1186/s40169-017-0175-0.
- Akinc, A., et al. (2019) The Onpattro story and the clinical translation of nanomedicines containing nucleic acid-based drugs. Nature Nanotechnology, 14(12), pp. 1084–1087,doi.org/10.1038/s41565-019-0591-y.
- Chung, Y. H., et al. (2020) COVID-19 Vaccine Frontrunners and Their Nanotechnology Design. ACS Nano, 14(10), pp. 12522–12537. doi.org/10.1021/acsnano.0c07197.
- Walsh, E. E., et al. (2020) Safety and Immunogenicity of Two RNA-Based Covid-19 Vaccine Candidates. New England Journal of Medicine, 383(25), pp. 2439–2450. doi.org/10.1056/NEJMoa2027906.
- Baden, L. R., et al. (2021) Efficacy and Safety of the mRNA-1273 SARS-CoV-2 Vaccine. New England Journal of Medicine, 384(5), pp. 403–416. doi.org/10.1056/NEJMoa2035389.
- Wilhelm, S., et al. (2016) Analysis of nanoparticle delivery to tumours. Nature Reviews Cancer, 1(5), p. 16014. doi.org/10.1038/natrevmats.2016.14.
- Cheng, Y. H., et al. (2020) Meta-Analysis of Nanoparticle Delivery to Tumors Using a Physiologically Based Pharmacokinetic Modeling and Simulation Approach. ACS Nano, 14(3), pp. 3075–3095. doi.org/10.1021/acsnano.9b08142.
- Anselmo, A C &Mitragotri, S (2019) Nanoparticles in the clinic: An update. Bioengineering & Translational Medicine, 4, p. 10143. doi.org/10.1002/btm2.10143.
- Zhong, H., et al. (2018) A Comprehensive Map of FDA-Approved Pharmaceutical Products. Pharmaceutics,10(4), p. 263. doi.org/10.3390/pharmaceutics10040263.
- (2019) The two directions of cancer nanomedicine. Nature Nanotechnology, 14(12), p. 1083. doi.org/10.1038/s41565-019-0597-5.
- Corbo, C., et al. (2016) The impact of nanoparticle protein corona on cytotoxicity, immunotoxicity and target drug delivery. Nanomedicine, 11(1), pp. 81–100. doi.org/10.2217/nnm.15.188.
- Caracciolo, G., et al. (2017) Biological Identity of Nanoparticles In Vivo: Clinical Implications of the Protein Corona. Trends in Biotechnology, 35(3), pp. 257–264. doi.org/10.1016/j.tibtech.2016.08.011.
- Donahue, N. D., et al. (2019) Concepts of nanoparticle cellular uptake, intracellular trafficking, and kinetics in nanomedicine. Advanced Drug Delivery Reviews, 143, pp. 68–96. doi.org/10.1016/j.addr.2019.04.008.
- Rampado, R., et al. (2020) Recent Advances in Understanding the Protein Corona of Nanoparticles and in the Formulation of “Stealthy” Nanomaterials. Frontiers in Bioengineering and Biotechnology, 8, p. 166. doi.org/10.3389/fbioe.2020.00166.
- Brenner, S & Lerner, R A (1992) Encoded Combinatorial Chemistry. Processing of the National Academy of Sciences of the USA, 89(12), pp. 5381– 5383. doi.org/10.1073/pnas.89.12.5381.
- Song, M & Hwang, G T (2020) DNA-Encoded Library Screening as Core Platform Technology in Drug Discovery: Its Synthetic Method Development and Applications in DEL Synthesis. Journal of Medicinal Chemistry, 63(13), pp. 6578–6599. doi.org/10.1021/acs.jmedchem.9b01782.
- Reiher, C. A., et al. (2021) Trends in Hit-to-Lead Optimization Following DNA-Encoded Library Screens. ACS Medicinal Chemistry Letters, 12(3), pp. 343–350. doi.org/10.1021/acsmedchemlett.0c00615.
- Chen, B., et al. (2020) Harnessing big ‘omics’ data and AI for drug discovery in hepatocellular carcinoma. Nature Reviews Gastroenterology & Hepatology, 17(4), pp. 238–251. doi.org/10.1038/s41575-019-0240-9.
- Matthews, H., et al. (2016) Omics“-Informed Drug and Biomarker Discovery: Opportunities, Challenges and Future Perspectives. Proteomes, 4(3), p. 28. doi.org/10.3390/proteomes4030028.
- Das, T., et al. (2020) Integration of Online Omics-Data Resources for Cancer Research. Frontiers in Genetics, 11, p. 578345. doi.org/10.3389/fgene.2020.578345.
- Conesa, A & Beck, S (2019) Making multi-omics data accessible to researchers. Scientific Data, 6, p. 251. doi.org/10.1038/s41597-019-0258-4.
- Chen, D. L., et al. (2012) Rapid Discovery of Potent siRNA-Containing Lipid Nanoparticles Enabled by Controlled Microfluidic Formulation. Journal of the American Chemical Society, 134(16), pp. 6948– 6951. doi.org/10.1021/ja301621z.
- Hajj, K A & Whitehead, K. A (2017) Tools for translation: non-viral materials for therapeutic mRNA delivery. Nature Reviews Materials, 2(10), p. 17056.doi.org/10.1038/natrevmats.2017.56.
- Kauffman, K. J., et al. (2015) Optimization of Lipid Nanoparticle Formulations for mRNA Delivery in Vivo with Fractional Factorial and Definitive Screening Designs. Nano Letters, 15(11), pp. 7300–7306 doi.org/10.1021/acs.nanolett.5b02497.
- Siegwart, D. J., et al. (2011) Combinatorial synthesis of chemically diverse core-shell nanoparticles for intracellular delivery. Processing of the National Academy of Sciences of the USA, 108(32), pp. 12996–13001. doi.org/10.1073/pnas.1106379108.
- Hao, J, et al. (2015) Rapid Synthesis of a Lipocationic Polyester Library via Ring-Opening Polymerization of Functional Valerolactones for Efficacious siRNA Delivery. Journal of the American Chemical Society, 137(29), pp. 9206– 9209. doi.org/10.1021/jacs.5b03429.
- Kumar, R., et al. (2020) Efficient Polymer-Mediated Delivery of Gene-Editing Ribonucleoprotein Payloads through Combinatorial Design, Parallelized Experimentation, and Machine Learning. ACS Nano, 14(12), pp. 17626–17639. doi.org/10.1021/acsnano.0c08549.
- Whitehead, K. A., et al. (2012) In Vitro-In Vivo Translation of Lipid Nanoparticles for Hepatocellular siRNA Delivery. ACS Nano, 6(8), pp. 6922–6929. doi.org/10.1021/nn301922x.
- Jain, P., et al. (2017) In-vitro in-vivo correlation (IVIVC) in nanomedicine: Is protein corona the missing link?.Biotechnology Advances, 35(7), pp. 889– 904. doi.org/10.1016/j.biotechadv.2017.08.003.
- Bruchez, M., et al. (1998) Semiconductor nanocrystals as fluorescent biological labels. Science, 281(5385), pp. 2013–2016. doi.org/10.1126/science.281.5385.2013.
- Chan, W C W & Nie, S M (1998) Quantum dot bioconjugates for ultrasensitive nonisotopic detection. Science, 281(5385), pp. 2016–2018. doi.org/10.1126/science.281.5385.2016.
- Mitchell, G. P., et al. (1999) Programmed assembly of DNA functionalized quantum dots. Journal of the American Chemical Society, 121(35), pp. 8122–8123. doi.org/10.1021/ja991662v.
- Han, M. Y., et al. (2001) Quantum-dot-tagged microbeads for multiplexed optical coding of biomolecules. Nature Biotechnology, 19(7), pp. 631–635. doi.org/10.1038/90228
- Nicewarner-Pena, S. R., et al. (2001) Submicrometer metallic barcodes. Science, 294(5540), pp. 137–141. doi.org/10.1126/science.294.5540.137
- Paunovska, K., et al. (2018) A Direct Comparison of in Vitro and in Vivo Nucleic Acid Delivery Mediated by Hundreds of Nanoparticles Reveals a Weak Correlation. Nano Letters, 18(3), pp. 2148–2157. doi.org/10.1021/acs.nanolett.8b00432
- Dahlman, J. E., et al. (2017) Barcoded nanoparticles for high throughput in vivo discovery of targeted therapeutics. Processing of the National Academy of Sciences of the USA, 114(8), pp. 2060–2065. doi.org/10.1073/pnas.1620874114.
- Gan, Z. B., et al. (2020) Nanoparticles containing constrained phospholipids deliver mRNA to liver immune cells in vivo without targeting ligands. Bioengineering & Translational Medicine, 5(3), p. e10161. doi.org/10.1002/btm2.10161.
- Lokugamage, M. P., et al. (2019) Constrained Nanoparticles Deliver siRNA and sgRNA to T Cells In Vivo without Targeting Ligands. Advanced Materials, 31(41), p. 1902251. doi.org/10.1002/adma.201902251.
- Adir, O., et al. (2020) Integrating Artificial Intelligence and Nanotechnology for Precision Cancer Medicine. Advanced Materials, 32(13), p. 1901989. doi.org/10.1002/adma.201901989.
- Paunovska, K., et al. (2019) Using Large Datasets to Understand Nanotechnology. Advanced Materials, i(43), p. 1902798. doi.org/10.1002/adma.201902798.
- Yaari, Z., et al. (2016) Theranostic barcoded nanoparticles for personalized cancer medicine. Nature Communications, 7, p. 13325. doi.org/10.1038/ncomms13325.
- Deng, Z. J., et al. (2013) Layer-by-Layer Nanoparticles for Systemic Codelivery of an Anticancer Drug and siRNA for Potential Triple-Negative Breast Cancer Treatment. ACS Nano, 7(11), pp. 9571–9584. doi.org/10.1021/nn4047925.
- Poon, Z., et al. (2011) Controlling in Vivo Stability and Biodistribution in Electrostatically Assembled Nanoparticles for Systemic Delivery. Nano Letters, 11(5), pp. 2096–2103. doi.org/10.1021/nl200636r.
- Morton, S. W., et al. (2013) The architecture and biological performance of drug-loadedLbL nanoparticles. Biomaterials, 34(21), pp. 5328–5335. doi.org/10.1016/j.biomaterials.2013.03.059.
- Dang, X. N., et al. (2016) Layer-by-layer assembled fluorescent probes in the second near-infrared window for systemic delivery and detection of ovarian cancer. Processing of the National Academy of Sciences of the USA, 113(19), pp. 5179–5184. doi.org/10.1073/pnas.1521175113.
- Correa, S., et al. (2020) Tuning Nanoparticle Interactions with Ovarian Cancer through Layer-by-Layer Modification of Surface Chemistry. ACS Nano, 14(2), pp. 2224–2237. doi.org/10.1021/acsnano.9b09213.
- Zhang, S., et al. (2008) Identification and characterization of ovarian cancer-initiating cells from primary human tumors. Cancer Research, 68(11), pp. 4311–4320. Available Online: https://cancerres.aacrjournals.org/content/68/11/4311.
- Ricardo, S., et al. (2011) Breast cancer stem cell markers CD44, CD24 and ALDH1: expression distribution within intrinsic molecular subtype. Journal of Clinical Pathology, 64(11), pp. 937–946. doi.org/10.1136/jcp.2011.090456.
- Choi, K. Y., et al. (2011) Smart Nanocarrier Based on PEGylated Hyaluronic Acid for Cancer Therapy. ACS Nano, 5(11), pp. 8591–8599. doi.org/10.1021/nn202070n.
- Boehnke, N., et al. (2020) Theranostic Layer-by-Layer Nanoparticles for Simultaneous Tumor Detection and Gene Silencing. AngewandteChemie International Edition, 59(7), pp. 2776–2783. doi.org/10.1002/anie.201911762.
- Velasco, V., et al. (2020) Microtechnology-based methods for organoid models. Microsystems & Nanoengineering, 6(1), p. 76. doi.org/10.1038/s41378-020-00185-3
- Chen, X., et al. (2021) Organ-on-a-chip platforms for accelerating the evaluation of nanomedicine. Bioactive Materials, 6(4), pp. 1012–1027. doi.org/10.1016/j.bioactmat.2020.09.022
- Jia, Z., et al. (2021) “Mucus-on-Chip”: A new tool to study the dynamic penetration of nanoparticulate drug carriers into mucus. International Journal of Pharmaceutics, 598, p. 120391. doi.org/10.1016/j.ijpharm.2021.120391.
- Shelton, S. E., et al. (2021) Engineering approaches for studying immune-tumor cell interactions and immunotherapy. iScience, 24(1), p. 101985. doi.org/10.1016/j.isci.2020.101985.
- Zhang, S., et al. (2021) Vascularized organoids on a chip: strategies for engineering organoids with functional vasculature. Lab on a Chip, 21(3), pp. 473–488. doi.org/10.1039/D0LC01186J.
- Wu, J. D., et al. (2018) Lab-on-chip technology for chronic disease diagnosis. npj Digital Medicine, 1(7)[P1] . doi.org/10.1038/s41746-017-0014-0.
- Hajal, C., et al. (2021) Biology and Models of the Blood-Brain Barrier. Annual Review of Biomedical Engineering, 23, pp. 359–384. doi.org/10.1146/annurev-bioeng-082120-042814.
- Perlman, R L (2016) Mouse models of human disease An evolutionary perspective. Evolution, Medicine, and Public Health, 1(1), pp. 170–176.doi.org/10.1093/emph/eow014.
- Alexis, F., et al. (2008) Factors affecting the clearance and biodistribution of polymeric nanoparticles. Molecular Pharmaceutics, 5(4), pp. 505–515. doi.org/10.1021/mp800051m.
- Longmire, M., et al. (2008) Clearance properties of nano-sized particles and molecules as imaging agents: considerations and caveats. Nanomedicine, 3(5), pp. 703–717. doi.org/10.2217/17435889.3.5.703.
- Brehm, M. A., et al. (2014) Generation of improved humanized mouse models for human infectious diseases. Journal of Immunological Methods, 410, pp. 3–17. doi.org/10.1016/j.jim.2014.02.011.
- Brehm, M. A., et al. (2010) Humanized mouse models to study human diseases. Current Opinion in Endocrinology, Diabetes and Obesity, 17(2), pp. 120–125. doi:10.1097/MED.0b013e328337282f.
- Robbins, G. R., et al. (2015) Analysis of human innate immune responses to PRINT fabricated nanoparticles with cross validation using a humanized mouse model. Nanomedicine: Nanotechnology, Biology and Medicine, 11(3), pp. 589–599. doi.org/10.1016/j.nano.2014.11.010.
- Dou, H., et al. (2006) Development of a macrophage-based nanoparticle platform for antiretroviral drug delivery. Blood, 108(8), pp. 2827–2835. doi.org/10.1182/blood-2006-03-012534.
- Zhou, J. H., et al. (2011) Systemic Administration of Combinatorial dsiRNAs via Nanoparticles Efficiently Suppresses HIV-1 Infection in Humanized Mice. Molecular Therapy, 19(12), pp. 2228–2238. doi.org/10.1038/mt.2011.207.
- Tian, H. M., et al. (2020) Humanized Rodent Models for Cancer Research. Frontiersin Oncology, 10, p. 1696. doi.org/10.3389/fonc.2020.01696.
- Kersten, K., et al. (2017) Genetically engineered mouse models in oncology research and cancer medicine. EMBO Molecular Medicine, 9(2), pp. 137–153. doi.org/10.15252/emmm.201606857.
- LamprehtTratar, U., et al. (2018) Transgenic Mouse Models in Cancer Research. Frontiers in Oncology, 8, p. 268. doi.org/10.3389/fonc.2018.00268.