The semiconductor industry is experiencing significant growth, and its proliferation has placed more urgency on the need to completely eliminate defects from the manufacturing chain.
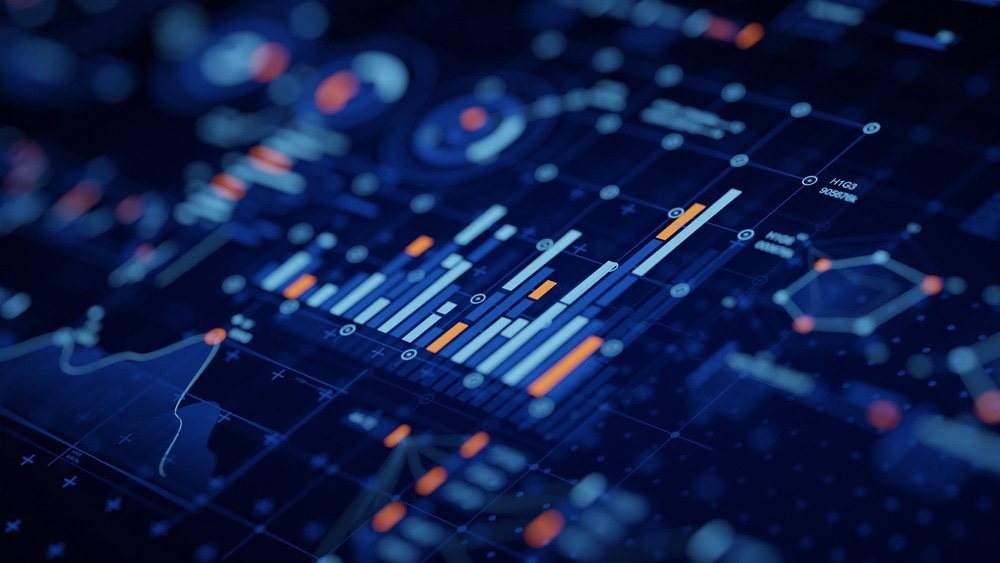
Image Credit: Chaosamran_Studio/Shutterstock.com
Currently, semiconductor defects are measured in terms of parts per billion. However, even the smallest defect rate in a manufacturing chain can have devastating consequences. For example, semiconductors are used in automobiles to control all kinds of systems, and malfunctions in these systems can have severe consequences.
As semiconductors make their way into more applications that potentially involve life and death, the need to completely eliminate defects in chip manufacturing becomes even more urgent. At the same time, greater and greater demand for semiconductors is increasing the need to produce more chips.
The use of semiconductor production data is now being seen as a critical component in addressing the dual needs for higher quality and greater production capacity. Gathering data in the semiconductor manufacturing chain does not pose a challenge. Every day, the industry collects vast amounts of data from its production equipment. Instead, the key challenge is using advanced analytics to transform that data into insights.
Data insights can be used in many different ways. At the design stage, insights could be used to improve performance and processing power. In the ramp stage, analytics can be used to decrease bring-up time. In the production stage, insights can boost quality, process productivity, and throughput.
While data analytics can be used to address problems or seize opportunities in each distinct phase, it is also important to develop holistic solutions that span the entire lifecycle. This is because a problem seen in one phase may have its roots in an earlier stage.
According to Accenture, there is a significant opportunity related to the great use of analytics in semiconductor manufacturing. A recent report from the company said the application of analytics to a production operation can cut machine downtime in half. The application of analytics and machine learning technology also has the potential to increase production yield by 5 to 15 percent.
The benefits of using data analytics in semiconductor manufacturing can be seen in several key areas:
- Identification of production metrics that have potential for improvement.
- Flagging of data outliers related to production issues.
- Root-cause analysis across the entire manufacturing chain.
- Consolidation of analytics across the manufacturing chain.
- Acceleration of speed-to-market, enabling more product customization.
Leveraging AI for Semiconductor Analytics
The emergence of AI and machine learning technologies means even more value can potentially be extracted from semiconductor data.
Many leading semiconductor manufacturers are currently using predictive AI to anticipate errors and equipment breakdown. As a result, these companies are seeing improvements in quality and yield.
One recent report by Deloitte showed how AI systems can analyze data almost 600 times faster than humans. Despite this staggering productivity improvement, only about 26% of manufacturers are currently using AI-powered analytics, according to a report from Gigaphoton.
Challenges and Opportunities for Advancing Analytics
While the adoption of data analytics in semiconductor manufacturing improves performance and efficiency, broader adoption of this technology is not going to happen overnight. Manufacturers looking to step up their use of analytics face key challenges in three areas: hardware, software, and data management.
With respect to hardware, semiconductor manufacturers use a patchwork of equipment, systems, sensors, and networks. The capabilities of each piece of hardware can vary significantly. While some can only log very basic descriptive data, some hardware can collect a wide range of data types.
The typical manufacturer also uses multiple software solutions, making it difficult to acquire deep insights. In fact, the Gigaphoton report revealed that software fragmentation is one of the biggest challenges to greater analytics adoption, with 73 percent of survey respondents saying they had to combine multiple analytics solutions.
A wide range of solutions could spell a well-rounded data analytics program, but some experts lament the fact that there is not an industry-standard, unified analytics platform. Therefore, analytics capabilities vary significantly from manufacturer to manufacturer.
Data management is another key challenge for the advancement of data analytics in the semiconductor manufacturing chain. While collecting data is not a problem for manufacturers, managing, storing, and accessing collected data is often done inefficiently and this makes insight generation more difficult. As with software, data management systems vary significantly from company to company. Some manufacturers manage their data with a single program, while others use massive databases that are constantly being updated by multiple platforms.
Advancing the use of analytics in any company will require focusing on these three key areas. A thorough assessment of these areas can reveal information gaps and any efficient processes. Fabscape recently released a five-stage maturity model meant to address these key areas.
- Initiate. Log data in a way that it can be easily viewed, accessed, and edited.
- Propagate. Establish multiple systems that are capable of logging data.
- Integrate. Combine logs from multiple sources into a single database for analysis and visualization.
- Automate. Set up automated systems for data collection and standardization.
- Radiate. Leverage AI technology to regularly analyze production data and generate both predictive and prescriptive insights.
The emergence of standards and models like the one from Fabscape indicates a growing desire to organize data analytics inside my conductor manufacturing. As these efforts mature, we will likely see the industry become more productive and move toward the aspirational goal of zero-defect production.
References and Further Reading
Accenture. (2023). Accenture Semiconductor Manufacturing Analytics. Available at: https://www.accenture.com/content/dam/accenture/final/a-com-migration/pdf/pdf-169/accenture-semiconductor-manufacturing-analytics-brochure.pdf#zoom=50. Accessed on November 6, 2023.
Minegishi, Y. (2023). How mature is your semiconductor manufacturing analytics?. Retrieved from https://www.embedded.com/how-mature-is-your-semiconductor-manufacturing-analytics. Accessed on March 9, 2023.
Deloitte. (2019 ). Semiconductors – the Next Wave: Opportunities and winning strategies for semiconductor companies. Available at: https://www2.deloitte.com/tw/en/pages/technology-media-and-telecommunications/articles/semiconductor-next-wave.html. Accessed on April 2023.
Disclaimer: The views expressed here are those of the author expressed in their private capacity and do not necessarily represent the views of AZoM.com Limited T/A AZoNetwork the owner and operator of this website. This disclaimer forms part of the Terms and conditions of use of this website.