The U.S. Department of Energy (DOE)’s Lawrence Berkeley National Laboratory (Berkeley Lab) has won three 2014 R&D 100 awards. This year’s winners include a fast way to analyze the chemical composition of cells, a suite of genetic tools to improve crops, and a bioinformatics platform for screening 3-D cell culture models.
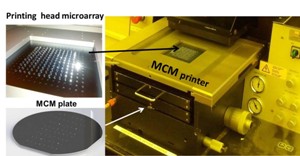
The technologies could lead to advances in biofuels, food crops, drug screening, and biomaterials, and a to better understanding of microbial communities, to name a few potential benefits.
Presented by R&D Magazine, the R&D 100 Awards recognize the year’s top 100 technology products from industry, academia, and government-sponsored research, ranging from chemistry to materials to biomedical breakthroughs. The three awards this year brings the total of Berkeley Lab’s R&D 100 wins to 73, plus two Editors’ Choice Awards.
A brief description of each of the winners follows.
Molecular-level Chemical Analyzer of Plant Cell Walls and Microbes
The Berkeley Lab Multiplex Chemotyping Microarray performs rapid chemical analyses of prospective biofuel crops and microbial communities by combining high-throughput micro-contact printing technology with high-fidelity vibrational spectroscopy and mass spectrometry. Its ability to rapidly identify the chemical composition and biological function in plant and animal cells is unparalleled.
The technology was developed by Hoi-Ying Holman of the Earth Sciences Division and her team of scientists, including ESD’s Liang Chen and Giovanni Birarda, as well as former ESD postdoc Sun Choi. It can be applied to biofuels, microbial ecological investigation, and medical research.
It enables precise deposition of samples and the detection of multiple chemicals within each sample at a throughput rate more than two orders of magnitude beyond existing methods. Samples can include plant particles, airborne particulates, and soil particles. It also includes microbes with sample volumes down to 10 picoliters, and mixtures of proteins or heterogeneous biomolecules with dimensions smaller than 50 nanometers.
Tissue-Specific Cell-Wall Engineering for Biofuels and Biomaterials
Tissue-Specific Cell-Wall Engineering for Biofuels and Biomaterials is a suite of precision genetic tools that will improve crops bred for production of food, biofuels, industrial polymers, and pharmaceuticals.
The technology was developed by Henrik Scheller and Dominique Loqué of the Physical Biosciences Division and the Joint BioEnergy Institute.
A common goal for biofuel crops is to reduce lignin, a tough polymer that is difficult to take apart and prevents access to the carbohydrates in biomass. Low-lignin traits can be transferred through gene-inactivation, but generally such plants grow poorly. The new approach restricts lignin to nutrient-carrying vessel cells, where its strength is essential for plant survival, while overall lignin levels stay low. The technology fine-tunes lignin by manipulating chemical signals that govern plant-cell metabolism. A key innovation, an artificial positive feedback loop, dramatically enhances this pinpoint control. Plants can be coaxed to make more cellulose and less xylan — also good for biofuels.
This synthetic biology platform can enhance drought-resistance, make cattle forage more nutritious, and even coax plants or fungi to yield high-value drugs and biomaterials.
BioSig3D
BioSig3D is a computational platform for high-content screening of three-dimensional cell culture models that are imaged in full 3-D volume. It is primarily used for the study of aberrant organization that is typically caused by cancer. It will also enable the evaluation and quantification of the effects of perturbagens, such as radiation exposures and environmental toxins, in a more effective model system.
The technology was developed by Bahram Parvin, Gerald Fontenay, Cemal Bilgin, Hang Chang, and Ju Han of the Life Sciences Division.
It provides an end-to-end solution for designing screening assays, provides novel algorithms for quantitative analysis of multicellular systems and their organization, enables visualization of complex raw and processed 3D spatial data, and integrates advanced bioinformatics analysis.
It is the first web-based imaging bioinformatics system that couples novel image analysis algorithms with 3-D data acquired using confocal or deconvolution microscopy. Adoption of this technology will lead to a more reliable and faster evaluation of therapeutic targets at lower prices, and improve understanding of high order cellular functions.