Modern nanotechnology products and methods require advanced nanomanipulation techniques to manufacture and work with nanoscale objects accurately, efficiently, and automatically. A recent study proposed a new nanomanipulation technique based on atomic force microscopy (AFM) combined with cutting-edge artificial intelligence (AI) deep learning programs.
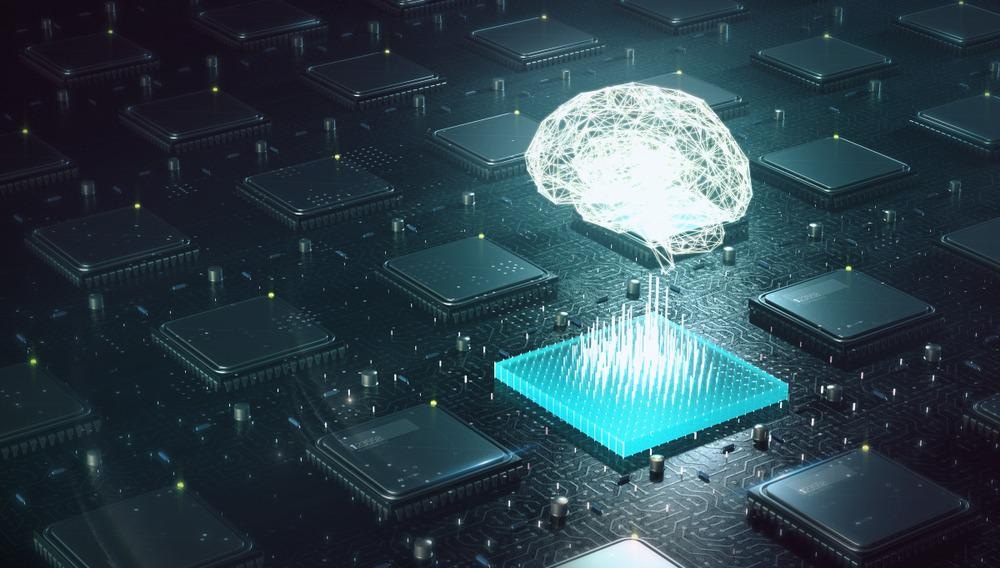
Image Credit: archy13/Shutterstock.com
AFM, Deep Learning, and Nanotechnology
Atomic force microscopy (AFM) is an advanced microscopy technique that employs an extremely sharp-tipped scanning probe attached to a cantilever to gather information about nanoscale details in the sample material. The probe reacts to atomic forces at the surface, and a detector records the resulting movement of the cantilever that the probe is attached to.
Deep learning algorithms contribute to artificial intelligence (AI) – computer processes that do not need constant user input to yield results. They instruct computers to process data and learn from it (known as training the algorithm) to inform their next steps.
Deep learning algorithms for computer vision train computer programs to “see” and recognize objects in image data. They can be applied to AFM images to instantly and reliably recognize target nanoscale features in the data.
AFM has been a mainstay of nanotechnology since its inception by IBM and Stanford University scientists in 1985. Researchers have used the technique to observe materials and components at the scale of a single atom.
Nanotechnology researchers use AFM because it provides a three-dimensional model of the sample’s surface at resolution levels below a single nanometer. Frictional, nanomechanical, electrical, magnetic, and thermal properties of nanostructures have all been examined using AFM.
Cutting-edge nanotechnology methods today combine AFM with computer vision algorithms to automate identification and classification processes. Deep learning enables these processes to happen dynamically, constantly feeding information to the algorithm which can then generate new instructions for the AFM probe – either to obtain new image data or to manipulate the nanoscale objects and features it was observing.
This cutting-edge approach can enable industrial-scale nanotechnology manufacturing to take place, as well as opening the door to numerous other research applications for nanotechnology and AFM.
Detecting Nanowires in AFM Images with Deep Learning
Nanomanipulation using AFM is a proven method for assembling nanoparticles into various patterns and nanoscale devices for nanotechnology. To automate nanomanipulation, making it efficient enough for extensive research purposes as well industrial applications, precise and automatic identification of nanoparticles on the substrate material is necessary.
In a recent study, researchers from Tianjin University, China, demonstrated how a deep learning algorithm could be applied to automatically detect nanowires in AFM image data. Then, their program separates nanowires into abstracted polygonal line segments that can be manipulated further using the AFM probe.
The study utilized two cutting-edge deep learning algorithms developed for computer vision tasks: You Only Look Once version 3 (YOLOv3) and a fully convolutional network (FCN). These combined to form an instance segmentation network – like a team of computer processes collaborating to identify and annotate different objects that are visible in the image data.
YOLOv3 is a deep learning algorithm for real-time object detection in still, moving, and live images. It was developed by computer scientists Joseph Redmon and Ali Farhadi in 2018, with the first version (YOLO) released in 2016.
YOLO established a new era in computer vision by unifying two tasks that were traditionally performed in separate functions: feature extraction and object localization. Previously, computer vision algorithms had to be trained to learn the common features of the objects they were looking for before being released back on the image data to find the target objects. YOLO enables dynamic image processing by finding features and using these to find objects simultaneously.
With this segmentation network, researchers could instruct computers to automatically segment any movable nanowires in the AFM data. The processed segmentation data was then processed again with follow-up image morphology and fitting algorithms.
In combination, these algorithms enabled the AI to detect the postures and positions of nanowires at a high level of abstraction. The program was capable of detecting nanowires with different morphologies with resolution to the nanometer.
In the testing dataset, the AI proved to be 90% reliable. The authors of the study also remarked that their detection results are less affected by varying image complexity when compared to available methods for nanowire detection in AFM images. They said that this demonstrated a good level of robustness for the algorithm.
Traditionally, morphology algorithms have been employed to detect nanoscale features in AFM images. Morphological image processing uses non-linear operations to process shapes and objects in image data, working based on pixels’ positions relative to one another.
The data-driven deep learning method described in this study advances these traditional morphology algorithms’ reach to include more complex nanowire features. It also makes automatic nanomanipulation more efficient. This makes it more of a viable option for industrial use while opening the door to more laboratory applications as well.
Further, the team said that the algorithm could be applied generally to all image sources – not just AFM data. It is also possible to transplant the deep learning method developed in their research to other detection programs looking for wire-shaped objects.
AI and Nanotechnology - How do They Work Together?
References and Further Reading
Almog, U. (2020). YOLOv3 Explained. Towardsdatascience.com. [Online] Available at: https://towardsdatascience.com/yolo-v3-explained-ff5b850390f.
Bai, H. and S. Wu (2021). Deep-learning-based nanowire detection in AFM images for automated nanomanipulation. Nanotechnology and Precision Engineering. Available at: https://doi.org/10.1063/10.0003218.
Pilkington, B. (2021). Making Atomic Force Microscopy Faster with Automated Imaging. AZO Optics. Available at: https://www.azooptics.com/Article.aspx?ArticleID=2080.
Redmon, J., S. Divvala, R. Girshick, and A. Farhadi (2016). You Only Look Once: Unified, Real-Time Object Detection. arXiv. Available at: https://arxiv.org/abs/1506.02640.
Disclaimer: The views expressed here are those of the author expressed in their private capacity and do not necessarily represent the views of AZoM.com Limited T/A AZoNetwork the owner and operator of this website. This disclaimer forms part of the Terms and conditions of use of this website.